MLOps, or DevOps for machine learning, is bringing the best practices of software development to data science.
You know the saying, “Give a man a fish, and you’ll feed him for a day… Integrate machine learning into your software development division and you’ll create a reactive development processes that will unite teams across your business and help achieve KPIs across all your automation projects!” You know that one, right?
MLOps, or DevOps for machine learning, is increasingly becoming a necessary skill set and focus for enterprises looking to leverage the benefits of machine learning in the real world. It is a practice for collaboration and communication between data scientists and engineers to improve automation to streamline repeatable machine learning end to end, including deploying to production environments.
Microsoft Azure Machine Learning, for example, provides enterprise-grade capabilities to accelerate the machine learning lifecycle. It empowers developers and data scientists of all skill levels to build, train, deploy, and manage models responsibly and at scale.
Learn more about MLOps in Azure Machine Learning.
The Problems
Unrealized Potential
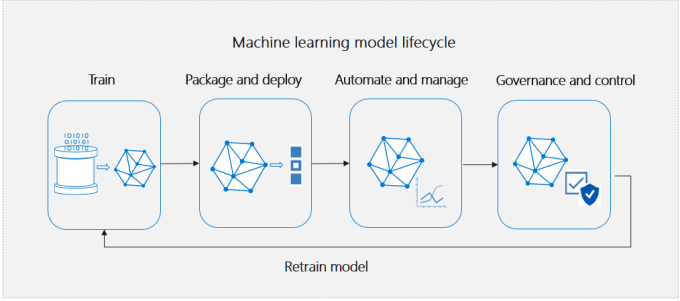
Many firms are racing to include machine learning in their applications to solve incredibly difficult business problems; however, for many, putting machine learning into production has proven even more difficult than simply finding good data scientists, data, and figuring out how to train the models. You can hire the most talented engineers in the world, with the most mature track record of machine learning development, and yet, all too often their sophisticated software solutions get held up in deployment—rendering them unproductive.
Frustrating Lag
Additionally, for many machine learning engineers, the process of deploying an already trained model can cause anxiety. Communication between operations teams and engineering teams requires incremental sign-offs and human facilitation which can take weeks or months. A relatively straightforward upgrade can feel like an insurmountable task, especially if the model has been improved by switching from one machine learning framework to another.
Fatigue
For managers, there’s nothing good to come of frustrated or underutilized engineers whose projects don’t make it to the finish line. Fatigue sets in, creativity stifles, and the motivation to come up with outstanding experiences for customers starts to dwindle.
Weak Communication
Data engineers, data scientists and software engineers seem to sit worlds apart from operations teams — and even if code manages to sneak out of development stages, there is rarely sufficient, streamlined support to bring your work to full production.
Lack of Foresight
Many data scientists who work on machine learning don’t have a concrete way of knowing that the models they train will work perfectly in production. Even if they write test cases and continually audit their work like QA or Unit Testers do in traditional software development, the types of data that may be encountered in production could be vastly different than the data that was used to train these models. Therefore, getting access to production telemetry to see how well the model performed against real world data and then use that data to improve the model and rapidly redeploy is extremely important. However, the lack of a streamlined CI/CD (continuous integration/ continuous delivery) process to get the new model into production is a major hindrance to realizing the value of machine learning.
Misused Talent
Data scientists are not IT engineers; they primarily focus on developing complex algorithms, neural network architectures and transformation of data, not the process of deploying microservices, network security, or other critical aspects of real-world implementations. MLOps lays the groundwork for multiple disciplines to with varied expertise to come together to infuse machine learning into real applications and services.
For the most part, these machine learning models are not intuitive for humans — their data-dependent functionality is built to grow beyond the day-to-day analysis traditionally used to monitor rule-based or database pattern matching. They require operations systems as sophisticated as the models themselves.
The Benefits of MLOps
Such is why more and more firms are investing resources into MLOps — to increase productivity and create trusted, enterprise-grade models. From innovative new businesses to large-scale public transportation divisions, teams are leveraging MLOps to make impactful change in their fields.
Open Communication
MLOps helps bring machine learning workflows to production by reducing friction between data science teams and operations teams. It opens up bottlenecks, especially those that form when complicated or niche machine learning models are siloed in development. MLOps systems establish dynamic, adaptable pipelines that enhance traditional DevOps systems in order to accommodate for ever-changing, KPI-driven models.
Repeatable Workflows
MLOps support and optimize upon models in order to allow automatic, streamlined changes. A model can journey its way toward processes that accommodate for data drift without six months of lag and email chains. MLOps consistently measure and order the behaviors of the model while it’s running. There’s a tandem sense of operation and iteration.
Governance / Regulatory Compliance
Perhaps even more incentivizing to firms is the ability for MLOps to aid in regulatory capacities. Machine learning guidelines are becoming increasingly stringent; take the GDPR in the EU or the Algorithmic Accountability Bill in New York City. MLOps systems are able to reproduce models in compliance and accordance with original standards. As consequent pipelines and models evolve, your systems continue to play by the rules.
Focused Feedback

You can also think of MLOps as a strong management defense against a slew of indecipherable analytics. During production, systems often face “alert storms” which can slow down training or even lead to failure. MLOps detect anomalies in machine learning development — helping engineers to more quickly understand what needs improving, and how severe the need really is.
Reduction in Bias
Additionally, MLOps can guard against certain biases in development — biases that can lead to under representing audiences, or exposing the company to legal scrutiny. Effective MLOps systems ensure that certain features within a data report do not outweigh others. MLOps support models as they adapt to their own evolution and drifts in data, creating dynamic systems that do not get pigeonholed in their reporting.
Generally speaking, MLOps is boosting the credibility, and reliability, and productivity of machine learning development. In essence, it’s helping machine learning to graduate into a respected, first-class asset of software development.
MLOps in Practice — Improving TransLink’s System in Vancouver
In the grand scheme of things, machine learning applications (and MLOps) are well-understood by a few — but their application often have broad impact. One such instance is a recent improvement at TransLink , who is the transit agency for Vancouver, Canada.
The problem was simple and common to many cities: everyone wanted to know exactly when the bus would show up. Vancouver’s bus system, which operates over 200 routes, including the busiest route in North America, and services over 2.5M residents, was no exception. As is true with any public transit agencies, the issue isn’t just setting schedules; it’s creating adaptive models that can react to delays and changing traffic patterns, anticipate predicted boarding times, and provide customers the most accurate possible time estimate.
To address growing customer demand for more accurate bus departures, TransLink partnered with Microsoft and T4G to build AI models that would better predict schedules. The teams created 16,000 completely independent learning models that running harmoniously in production with Azure. Together, the 16,000 models can automatically predict accurate departure and arrival times for all buses on the road.
But how did they do it? TransLink was used to working with traditional software development technologies from Microsoft. As they developed their new AI solution, they folded traditional DevOps practices with new MLOps options available through Azure Machine Learning. By transitioning their operations to a system that caters to machine learning, the team was able to deploy and monitor highly sophisticated models.
“Our data scientists could review the automated model training results before deployment and build in a data drift system to automatically look for deviations that might negatively impact our solution,” says Sze-Wan Ng, Director Analytics & Development at Translink.
MLOps also allowed the TransLink team to do the following:
- Set up automated build and release pipelines so that the model training and deployment processes could be automated.
- Have an approval process where data scientists can review automated model training results before deployment.
- Build a data drift system to automatically look for deviations in distribution of current data sources compared to them during model training time.
- Integrate data drift system to the build and release pipelines so upon detection of data distribution deviations, the model training and deployment pipelines can be triggered automatically
TransLink noted that the changes “improvement between predicted and actual bus departure times of 74%,” — a new horizon that the entire Vancouver community can celebrate.
Applying MLOps
In both the public sector and the private sector, MLOps is boosting the credibility, reliability, and productivity of machine learning development. In essence, it’s helping machine learning to graduate into a respected, first-class asset of software development.
Eric Boyd, CVP Azure AI Platform, explains that MLOps will help to accelerate the next step in creating useful machine learning. “We have heard from customers everywhere that they want to adopt ML but struggle to actually get models into production,” Boyd explains. “With the new MLOps capabilities in Azure Machine Learning, bringing ML to add value to your business has become better, faster, and more reliable than ever before.”
So how do you integrate MLOps into your production path? What are the end-to-end steps in MLOps? For the most part you can think about them in a handful of stages: Collaborate, Build, Test, Release, and Monitor. The workflow joins together app developers and data scientists into the same, automated system — one that continually audits and manages model interpretability over time.
Many firms are actively utilizing the powers of MLOps software already to optimize the performance and lifespan of their models. On top of that, the companies are able to share their knowledge and successes in an open-source environment, allowing broader knowledge about machine learning operation to spread and be adopted more quickly.
In addition to public transportation, various fields in healthcare, engineering, safety, and manufacturing have engaged MLOps to improve their machine learning development practices. From models that automatically recognize certain types of medication to models that anticipate the effects of earthquakes, autonomous (and deployed!) systems are yielding real-world impact with the assistance of MLOps.
In many ways, the risks are exponential in allowing the gap between data scientists and operations teams to grow further. Software that engages machine learning is growing at an incubated, rapid pace — the farther down the road it gets without grounded operational procedures, the less and less likely it will be considered for enterprise-grade application. There’s precedent for this type of growth: “database administrators,” for example, came about at the rise of early databases. The entirety of the world of DevOps is a reaction to systematic organization needed around quickly developing software.
Like successful DevOps initiatives before them, the MLOps programs of today help to unify disparate teams taking on challenging, innovative projects. Organizations of all types are finding the control provided by a successful MLOps strategy can lead to more efficient, productive, accurate, and trusted models in the long run. It’s all about doing a little housework before you dive into your homework. You can’t get work done in a siloed mess, even if you’re training a machine to do it. The time has come to embrace the potential of effective machine learning.
Get started with MLOps in Azure Machine Learning for free.